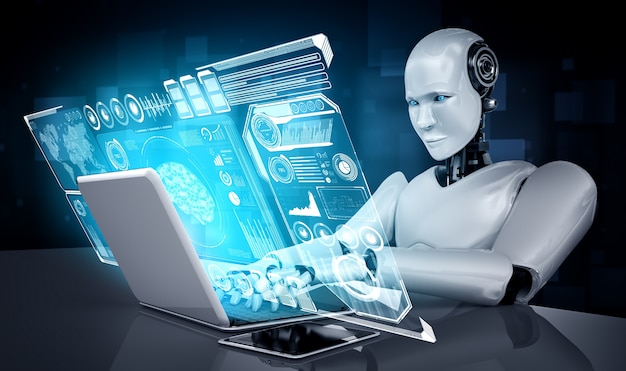
Building Blocks of Artificial Intelligence
The building blocks of artificial intelligence They are the fundamental components and core of AI systems, essentially the main pillars that enable machines to mimic human intelligence. These building blocks in AI form the core functions that allow AI to learn, understand, see, reason, and act. Think of them as the essential elements, not unlike the fundamental units in construction or the basic structures of artificial neural networks.
In essence, these building blocks of artificial intelligence – Machine Learning, Deep Learning, Natural Language Processing, Computer Vision, Expert Systems, Robotics, Knowledge Representation and Reasoning, Reinforcement Learning, Optimization, and Planning – are the core of what makes AI work. Machine Learning algorithms learn patterns from data to make predictions, and their importance lies in enabling AI to adapt and improve, though it’s unlikely to fully replace nuanced human decision-making
. Deep Learning, with its artificial neural networks, powers applications like image recognition and improves AI by automatically learning complex features, positioning it as a significant aspect of AI’s future. Natural Language Processing enables chatbots to understand user input, faces challenges in comprehending the complexities of human language, and is still far from truly understanding human emotions.
Computer Vision is used across industries for tasks like object detection, achieving varying levels of accuracy, and augmenting rather than entirely replacing human visual skills. Expert Systems solve problems by applying rule-based knowledge, with examples in healthcare and continued relevance in structured domains.
AI is transforming Robotics by enabling autonomous and adaptive behavior, allowing robots to perform increasingly human-like tasks while raising risks concerning automation and safety.
Knowledge Representation and Reasoning deal with how AI stores and processes information using techniques like semantic networks, crucial for enabling AI to “think” logically
. Reinforcement Learning, used in areas like autonomous driving, differs from supervised learning by learning through trial and error, allowing robots to learn independently.
Optimization is critical for the accuracy and speed of machine learning models, employing techniques like gradient descent. Finally, Planning allows AI systems to sequence actions to achieve goals, as seen in robotics navigation and automated scheduling.
Foundational Elements of AI
What is the crucial role of building blocks of artificial intelligence in understanding their individual roles and significance in creating intelligent systems?
1. Machine Learning (ML): Teaching Computers
Imagine showing a child many pictures of cats and dogs, and eventually, they learn to tell the difference on their own. Machine Learning works similarly. Instead of explicit instructions for every situation, we feed computers vast amounts of data, and they learn patterns and make predictions or decisions based on that learning.
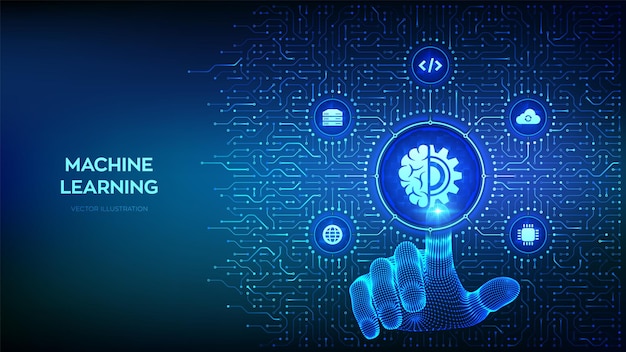
- What are the types of machine learning algorithms? There are several ways machines can learn. Supervised learning is like the cat and dog example – we provide labeled data (this is a cat, this is a dog) and the algorithm learns to classify new data. Unsupervised learning is like giving the computer a pile of mixed objects and asking it to find natural groupings or patterns without prior labels. Reinforcement learning (which we’ll discuss later as its own pillar) is like learning through trial and error, receiving rewards for good actions and penalties for bad ones.
- Why is machine learning important for AI development? ML is the engine that allows AI systems to adapt, improve, and make intelligent decisions without constant human programming. It’s what enables AI to handle complex and ever-changing real-world scenarios.
- Can machine learning replace human decision-making? In many specific, data-driven tasks, ML can outperform humans in speed and accuracy. However, human decision-making often involves creativity, emotional intelligence, ethical considerations, and understanding nuanced contexts that are currently difficult for machines to replicate fully. ML can augment and assist human decision-making, but a complete replacement in all domains is still a significant challenge.
2. Deep Learning (DL): Unleashing the Power of Artificial Neural Networks
Think of Deep Learning as a more sophisticated version of Machine Learning, inspired by how the human brain works. It uses complex structures called artificial neural networks with many layers (“deep”) to analyze information in a hierarchical way, learning intricate patterns from vast amounts of data.
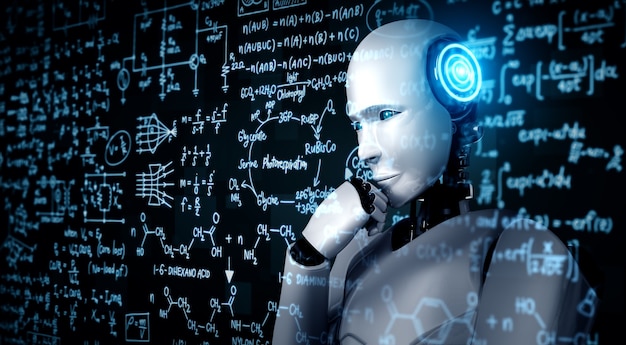
- What are common applications of deep learning? You encounter deep learning every day! It powers highly accurate image and speech recognition (like in your smartphone), natural language understanding in advanced chatbots, and complex pattern recognition in areas like fraud detection and medical diagnosis.
- How does deep learning improve AI systems? The “deep” structure allows these networks to automatically learn complex features from raw data without explicit human feature engineering. This makes them incredibly powerful for tackling intricate problems that traditional ML algorithms struggle with.
- Is deep learning the future of artificial intelligence? Deep learning is a dominant force driving many recent AI breakthroughs and is likely to remain crucial for future advancements, especially in areas requiring the processing of complex, unstructured data like images, audio, and text. However, it’s not the only future; other AI approaches and hybrid models will continue to play important roles.
3. Natural Language Processing (NLP): Bridging the Gap Between Humans and Machines
Natural Language Processing (NLP) is all about enabling computers to understand, interpret, and generate human language, whether spoken or written. It’s the key to making machines communicate with us in a way that feels natural.
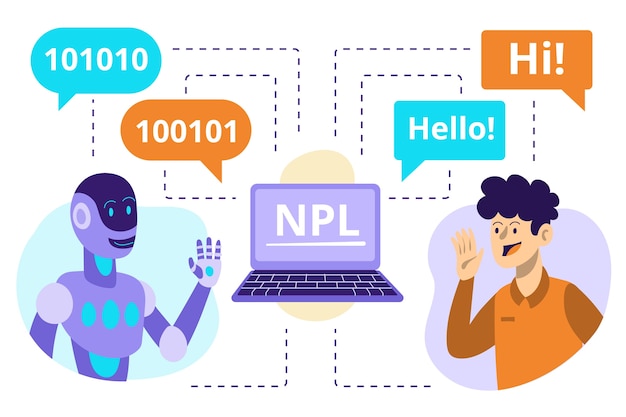
- How does natural language processing work in chatbots? In chatbots, NLP algorithms first analyze the user’s text input to understand their intent (what they are asking). Then, the system uses NLP to formulate a relevant and coherent response in human-like language.
- What are the challenges in natural language processing? Human language is incredibly complex and nuanced. Challenges include dealing with ambiguity (words having multiple meanings), sarcasm, context-dependent meaning, different languages and dialects, and the ever-evolving nature of language.
- Can NLP make machines understand human emotions? While NLP can analyze text and speech for indicators of emotion (sentiment analysis), truly understanding the depth and complexity of human emotions, with all their cultural and personal variations, is a significant ongoing challenge. Machines can recognize patterns associated with emotions but don’t “feel” them.
4. Computer Vision (CV): Giving Machines the Power of Sight
Computer Vision aims to equip computers with the ability to “see” and interpret the visual world from images and videos, much like humans do with their eyes and brains.
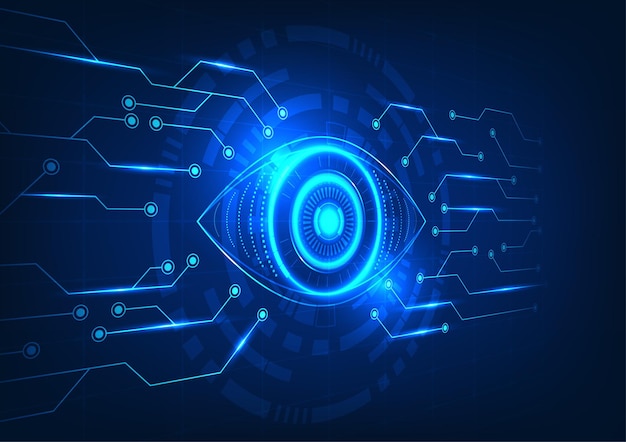
- What industries are using computer vision today? The applications are vast! Autonomous vehicles use CV to navigate roads, healthcare uses it for analyzing medical images, manufacturing employs it for quality control, security systems utilize facial recognition, and retail uses it for inventory management and customer behavior analysis.
- How accurate is computer vision technology? Accuracy varies greatly depending on the specific task and the quality of the images or videos. For well-defined tasks like recognizing simple objects under good lighting conditions, accuracy can be very high, sometimes even surpassing human capabilities. However, accuracy can decrease significantly with complex scenes, poor lighting, or occluded objects.
- Can computer vision replace human visual skills? In many repetitive and detail-oriented visual tasks, CV can be more accurate and efficient than humans. However, human vision excels in understanding context, recognizing novel objects, and making complex interpretations in ambiguous situations, areas where CV is still actively being developed.
5. Expert Systems (ES): Capturing Human Knowledge in Code
Expert Systems are designed to mimic the decision-making abilities of a human expert in a specific, well-defined area. They work by using a knowledge base filled with rules and facts provided by human experts to solve problems and provide advice.
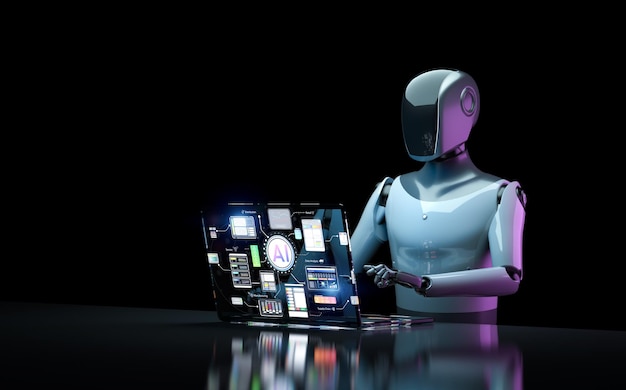
- How do expert systems solve complex problems? They use an “if-then” rule-based approach. The system takes in information, matches it against the rules in its knowledge base, and then applies those rules to reach a conclusion or recommendation.
- What are examples of expert systems in healthcare? Early expert systems were used for medical diagnosis, helping doctors identify potential illnesses based on patient symptoms and medical history. They could also assist in suggesting treatment plans.
- Are expert systems still relevant today? While the term “expert system” might not be as trendy as “machine learning,” the underlying principles of encoding knowledge and using rules are still valuable and are often integrated into more complex AI systems. They are particularly useful in domains where knowledge is well-structured and explainable.
6. Robotics: Bringing AI into the Physical World
Robotics combines AI with engineering to create intelligent machines that can perform physical tasks in the real world. AI provides the “brain” for robots, enabling them to perceive their environment, make decisions, and act autonomously.
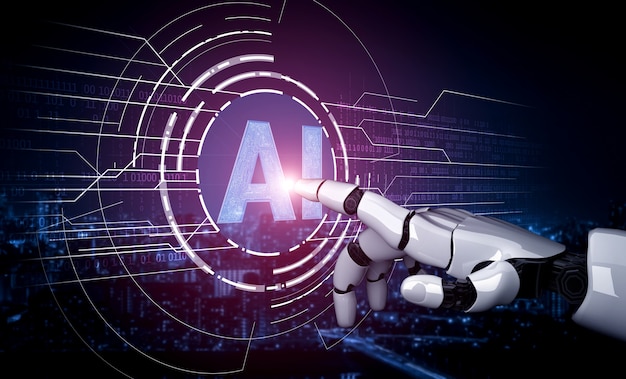
- How is AI transforming robotics? AI allows robots to move beyond pre-programmed tasks and adapt to new situations, learn from experience, collaborate with humans, and perform more complex and unstructured tasks in dynamic environments.
- Can robots with AI perform human-like tasks? Robots are becoming increasingly capable of performing tasks that require dexterity, problem-solving, and even some forms of social interaction. However, replicating the full range of human skills, including creativity, emotional intelligence, and adaptability in all situations, remains a significant challenge.
- What are the risks of AI in robotics? Concerns include the potential for job displacement through automation, safety issues with autonomous robots operating in human environments, and the ethical implications of increasingly sophisticated and potentially weaponized robots.
7. Knowledge Representation and Reasoning (KRR): Giving AI the Ability to Think
Knowledge Representation and Reasoning (KRR) focuses on how AI systems can store and process knowledge in a structured way so they can understand the world and draw logical conclusions. It’s about giving AI the ability to “think” and reason.
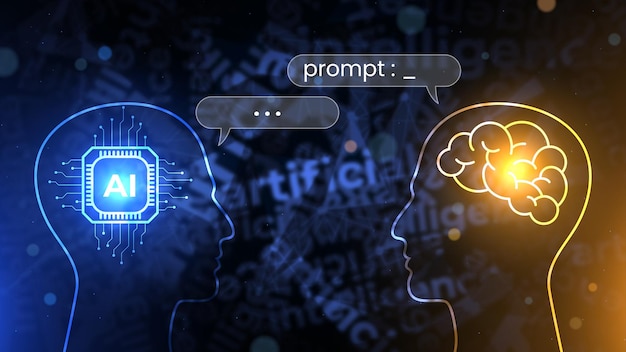
- How does AI store and process knowledge? AI uses various techniques to represent knowledge, such as semantic networks (representing relationships between concepts), ontologies (formal frameworks of knowledge), and logical rules. It then uses reasoning techniques like inference to derive new knowledge from existing information.
- What are the techniques for knowledge representation? Common techniques include using structured data formats, creating knowledge graphs that show connections between entities, and employing logical formalisms to represent facts and rules.
- Why is reasoning important in artificial intelligence? Reasoning allows AI to go beyond simply recognizing patterns and to understand the underlying relationships and implications of information. This is crucial for tasks like problem-solving, planning, and making informed decisions in complex situations.
8. Reinforcement Learning (RL): Learning by Doing and Getting Feedback
Reinforcement Learning (RL) is a type of machine learning where an agent learns to behave in an environment by performing actions and receiving rewards or penalties.
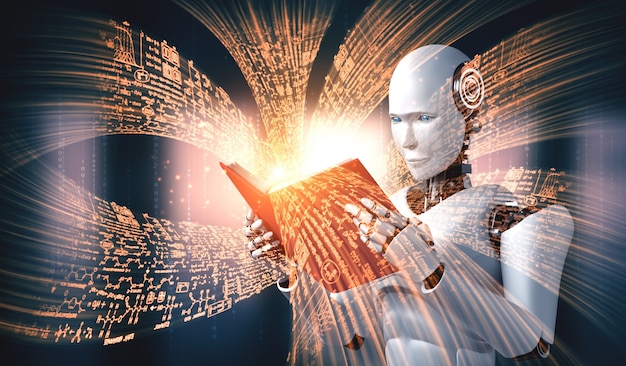
- Where is reinforcement learning used in real life? RL is used in training autonomous vehicles to navigate traffic, teaching robots complex motor skills, optimizing game-playing AI (like those that can beat humans at Go), and personalizing recommendations in online services.
- How does reinforcement learning differ from supervised learning? In supervised learning, the AI is given labeled examples of correct actions. In RL, the AI learns through trial and error based on the consequences of its actions (rewards or penalties) without explicit instructions on the “right” answer.
- Can reinforcement learning help robots learn on their own? Yes, RL enables robots to learn complex behaviors autonomously through interaction with their environment. They can explore different actions and learn which ones lead to the best outcomes without needing pre-programmed instructions for every possible scenario.
9. Optimization: Making AI Systems Efficient and Accurate
Optimization in AI involves finding the best possible set of parameters or strategies for an AI model to achieve its goals efficiently and accurately. Think of it as fine-tuning an engine to get the best performance.
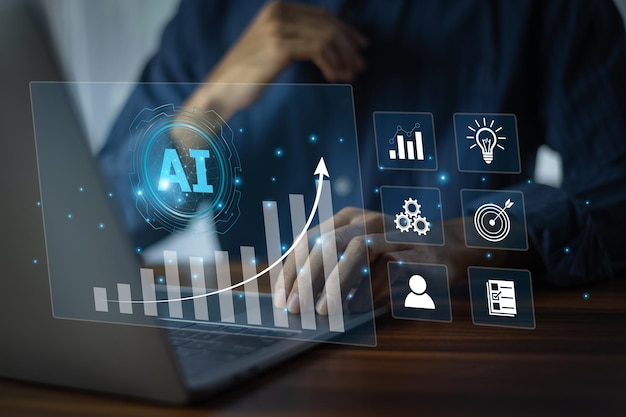
- Why is optimization critical for machine learning models? Optimization algorithms are used to train machine learning models by adjusting their internal parameters to minimize errors and improve their ability to make accurate predictions or classifications. Without effective optimization, models might be inaccurate or take too long to learn.
- What are common optimization techniques in AI? Techniques include gradient descent and its variants (like Adam and RMSprop), evolutionary algorithms, and various mathematical optimization methods.
- How does optimization affect AI accuracy and speed? Effective optimization leads to more accurate AI models because they can better learn the underlying patterns in the data. It also affects the speed of training (how quickly the model learns) and the efficiency of the model during deployment (how quickly it can make predictions).
10. Planning (AI Planning): Charting the Course for Intelligent Action
Planning in AI focuses on developing sequences of actions that an intelligent agent can take to achieve a desired goal. It’s about giving AI the ability to strategize and figure out the steps needed to reach a desired outcome.
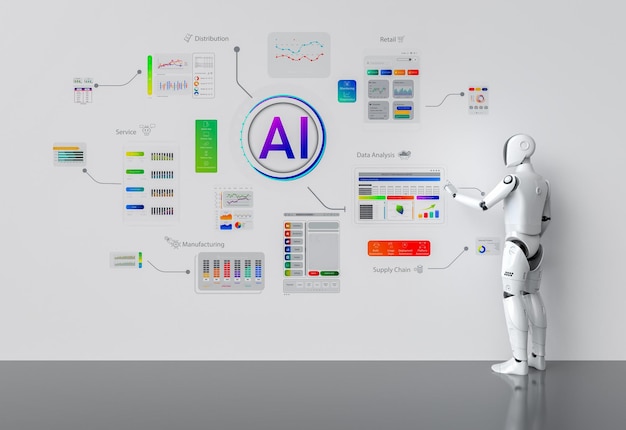
- How do AI systems plan actions to achieve goals? AI planning algorithms take into account the current state of the environment, the desired goal, and the available actions. They then search for a sequence of actions that will transform the initial state into the goal state.
- What are examples of AI planning in robotics? A robot navigating a warehouse to pick up and deliver items uses AI planning to determine the optimal path and sequence of movements. Similarly, robots performing complex assembly tasks in manufacturing rely on planning to coordinate their actions.
- What is automated planning and scheduling in AI? This involves AI systems automatically generating plans and schedules for complex tasks, such as managing production lines, coordinating logistics, or scheduling appointments. It aims to optimize efficiency and resource utilization.